As we stand on the brink of an AI revolution, action-driven AI agents are set to take center stage. From sophisticated chatbots to intelligent agents capable of managing emails and scheduling appointments, we are entering a transformative agentic era. To facilitate a deeper understanding of AI agents, this article will explore various types of AI agents, along with examples of leading companies that have integrated these innovations into their offerings. Let’s get started.
1. Understanding Simple Reflex Agents
Beginning with the most fundamental AI agents, Simple Reflex Agents respond to immediate inputs following an ‘if-then’ rule. For instance, a thermostat activates heating when the temperature drops below a specified threshold; this is an example of a reactive system acting on the present condition.
However, these agents are limited by their inability to remember previous states or to anticipate future conditions; they operate solely based on the current input. Simple Reflex Agents are efficient only in fully observable environments, where all necessary information is readily available.
2. Exploring Model-Based Reflex Agents
Model-Based Reflex Agents enhance upon their simpler counterparts by incorporating an internal representation of their environment. This allows them to maintain a memory of previous actions and their effects. For instance, a self-driving car navigates traffic by remembering the positions of nearby vehicles, even as they move.
These agents can operate in partially observable settings, where they utilize an internal model to predict subsequent actions based on past experiences and current observations.
3. The Functionality of Goal-Based Agents
As the name suggests, Goal-Based Agents are designed with outcomes in mind. They calculate the future actions that will lead to a desired objective, engaging in actions that optimize their path towards a goal. For example, a GPS system evaluates various routes based on distance, travel time, and traffic conditions, selecting the most efficient path to reach a destination.
4. Understanding Utility-Based Agents
Utility-Based Agents, a subset of Goal-Based Agents, evaluate varied potential outcomes rather than adhering to a strict binary of success or failure. These agents assign numerical values to possible actions based on complex preferences, ultimately selecting the action that maximizes their utility score.
For example, in an AI-driven trading system, the agent aims to maximize profit while considering user risk tolerance and current market conditions. Hence, Utility-Based Agents evaluate alternatives to find the optimal action.
5. Advancements with Learning Agents
Learning Agents improve over time by assimilating knowledge from past interactions, enabling them to adapt to novel environments. These agents include a “critic” component that provides feedback on their performance, further refining their actions.
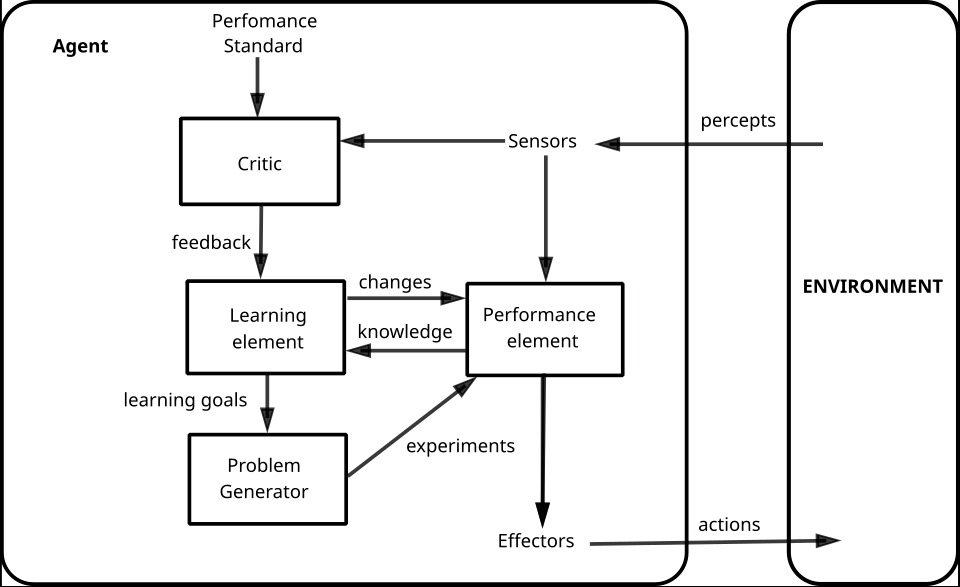
A practical example is a spam filter: initially set with basic rules, it adjusts its responses based on user feedback, marking spam emails automatically after receiving input on user preferences.
6. The Role of Hierarchical Agents
Hierarchical Agents decompose complex goals into sub-goals, effectively managing intricate tasks requiring multi-step solutions. By organizing tasks hierarchically, higher-level agents oversee strategy while lower-level agents handle specific tasks.
For instance, when programming an AI to prepare dinner, a high-level agent might plan the sequence of making pasta and sauce, breaking tasks down into manageable actions, like boiling water and serving sauce on the pasta.
7. Overview of Multi-Agent Systems
Multi-Agent Systems (MAS) comprise multiple autonomous agents collaborating to achieve a common goal. These agents interact, negotiate, and coordinate actions while retaining their independent decision-making capabilities.
In a supply chain example, one agent tracks inventory, while another generates procurement reports based on inventory demands, and a logistics agent finds the optimal shipping route.
Current AI Agents from Leading Technology Firms
Numerous advanced AI agents are currently available in the market. Companies like OpenAI, Google, Microsoft, Anthropic, and Salesforce are actively developing innovative AI agents and frameworks to harness the potential of agentic AI. Here’s a closer look.
OpenAI’s Innovations
OpenAI introduced the Operator AI agent, a consumer-centric tool designed to automate online tasks. This agent interacts with web browsers to fill forms, book flights, and order groceries, though manual input is still needed for payments and CAPTCHAs.
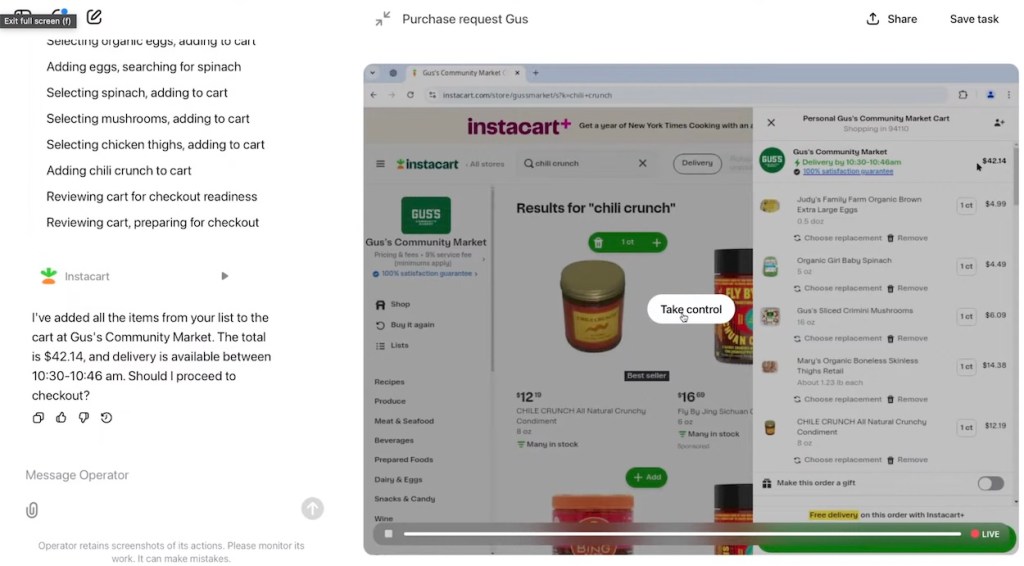
Falling under the categories of Goal-based and Learning agents, the Operator adapts through interaction. Additionally, OpenAI’s Deep Research agent performs intricate multi-step research tasks, blending elements of Goal-based, Learning, and Hierarchical agents by breaking down tasks into smaller components.
OpenAI’s latest systems, o3 and o4-mini, are designed as agentic platforms that interact with tools like web searches, Python interpreters, and more; these systems function as both Model-based and Goal-based agents.
The Codex CLI tool enables developers to read, modify, and execute code directly from the Terminal. This tool is classified as a Goal-based agent, integrated with Learning capabilities for continuous improvement.
Google’s Developments
Google has revealed the Deep Research AI agent on Gemini, which mirrors OpenAI’s agent by gathering and synthesizing information for comprehensive reports. This agent is classified as both Goal-based and Learning.
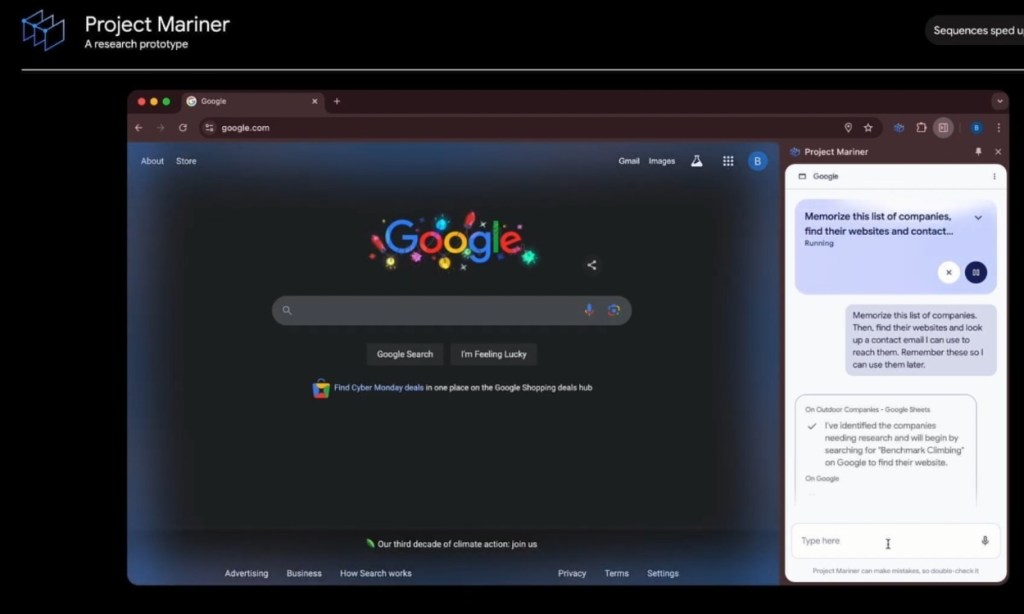
Additionally, Google is developing Project Mariner, similar to OpenAI’s Operator Agent, capable of automating tasks in Chrome. Currently in testing with select users, it promises to enhance productivity upon release.
Furthermore, Google has introduced the Agent2Agent (A2A) protocol, a framework that facilitates communication between multiple AI agents, laying the groundwork for efficient Multi-Agent Systems.
Anthropic’s Initiatives
Similar to OpenAI, Anthropic has created a Computer Use AI agent in beta that interacts with desktop environments, performing actions beyond web browsers, including file operations at the OS level.
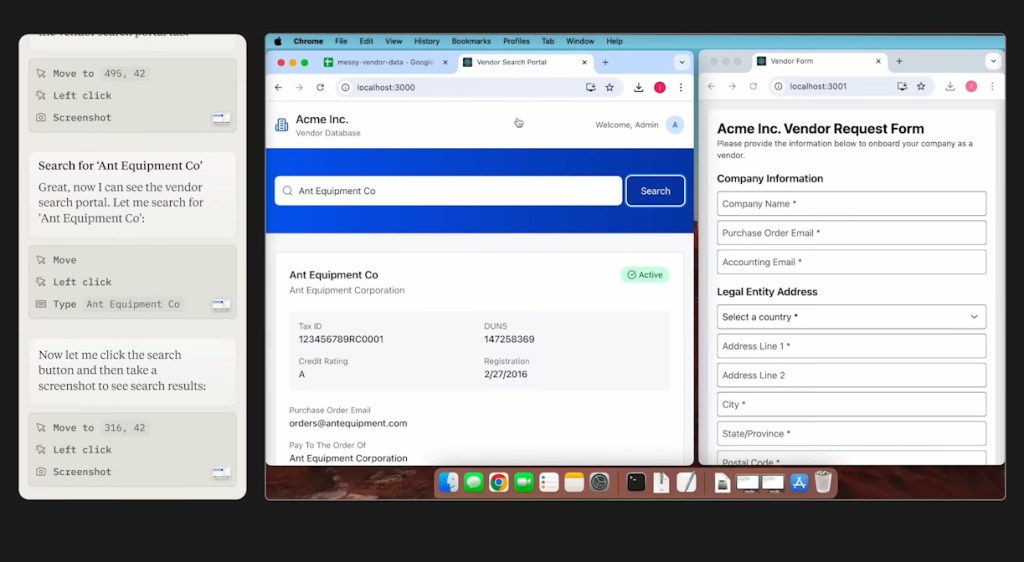
Anthropic also released a comprehensive Research tool integrated with Workspace, allowing connections to Gmail, Calendar, and Drive for insights extraction. Another addition is Claude Code, a coding tool that interacts within the Terminal, editing files and executing tests. Both belong to the Goal-based agent category.
Lastly, Anthropic has developed the Model Context Protocol (MCP), which standardizes communication between AI models and external data sources, enhancing reliability and interoperability.
Microsoft’s Contributions
Microsoft has introduced various AI agents within its Copilot framework. The Deep Research agent in Copilot can execute multi-step research, crafting detailed reports on specified topics. The Copilot Actions feature can facilitate online transactions, book reservations, and make purchases, although it is limited to partner websites.
Moreover, Microsoft’s Computer Use AI agent in Copilot Studio operates directly with websites and desktop applications to perform actions without specialized APIs. Security Copilot, another innovative agent, assists with critical tasks like phishing alerts and data security management.
Enterprises can harness various Copilot agents, and even create customized AI agents tailored to specific workflows via Copilot Studio, integrating MCP servers and external resources.
Salesforce’s Agentforce
Salesforce has developed Agentforce, allowing businesses to deploy customizable autonomous AI agents. This platform enables users to manage multiple agents designed for lead generation, sales enhancement, and marketing optimization.
Unlike Microsoft’s Copilot, Salesforce emphasizes the autonomy of its Agentforce agents, which can respond to events or triggers to perform tasks such as updating records, sending emails, scheduling meetings, and resolving cases.
In summary, these AI types and the current offerings from prominent tech firms reflect the potential of AI agents in reshaping industries. As technology advances, AI agents will undoubtedly become integral to both consumer and enterprise experiences.
Frequently Asked Questions About AI Agents
What Are AI Agents?
AI agents are autonomous programs designed to perform tasks on behalf of users, utilizing various AI techniques to process information, react to stimuli, and learn from experiences.
How Do AI Agents Work?
AI agents operate by receiving inputs from their environment, processing this information using predefined algorithms, and performing actions that achieve specific outcomes or goals.
What Are the Different Types of AI Agents?
AI agents can be categorized as Simple Reflex Agents, Model-Based Reflex Agents, Goal-Based Agents, Utility-Based Agents, Learning Agents, Hierarchical Agents, and Multi-Agent Systems, each serving different functions and use cases.
What Are Real-World Examples of AI Agents?
Examples include OpenAI’s Operator AI agent for automated online tasks, Google’s Deep Research AI agent for synthesizing information, and Salesforce’s Agentforce for customizable business applications.
Where Are AI Agents Used?
AI agents are utilized across various industries, including customer service, finance, logistics, healthcare, and more, streamlining operations and enhancing user experiences.